GPT Detectors Biased Against Non-Native English Writers, Study Finds
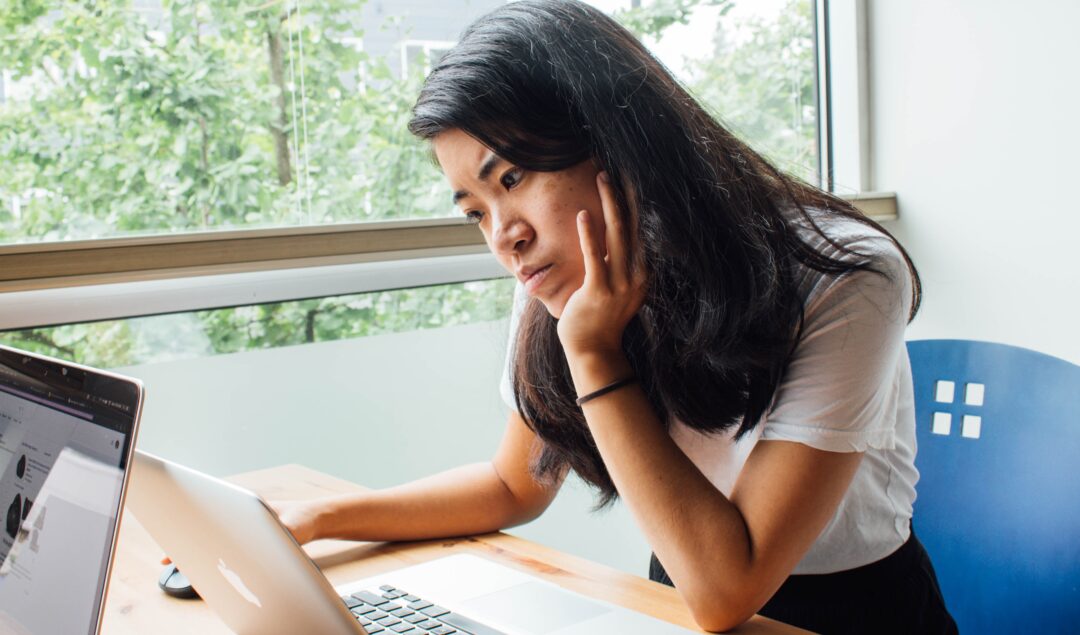
GPT detectors are biased against non-native English writers, a new study by researchers from Stanford University has found.
From the Balenciaga Pope to fake Trump arrest photos, the spread of AI-generated content is driving the demand for effective ways to detect AI-made content.
However, a recent study has found that GPT detectors often mistake non-native English writing for AI-generated text, while correctly identifying native English writing.
In tests, the detectors were near-perfect at analyzing US college admission essays but incorrectly classified over half of the Test of English as a Foreign Language (non-native English) essays as AI-generated. This could have potentially damaging consequences for non-native speakers where GPT detectors are used in evaluative or educational settings.
Non-native English writers tend to use less complex and diverse words, sentence structure, and grammar, so the researchers prompted a GPT-4 model to “enhance the word choices to sound more like that of a native speaker.”
When they ran this new “enhanced” essay through GPT detectors, there was a huge reduction in misclassification.
Read: Meet Edward Tian, The Man Fighting Against ChatGPT Misuse
The study has some limitations. The researchers suggest that larger and more diverse datasets are necessary to validate the findings. Additionally, newer GPT models may have fewer biases than those tested in the study.
Still, the study is an important step in understanding the biases present in these detectors.
The researchers recommend using more advanced techniques, such as second-order perplexity methods and watermarking techniques, to more accurately distinguish between AI-generated and human text.
“We should be *very cautious* when using detectors to classify if text is written by AI or human,” assistant professor at Stanford University and study co-author, James Zou, wrote on Linkedin.
“We find a general trend that more literary language are classified by detectors as more “human”. This leads to bias/false positives against non-native speakers. It’s also easy for #AI to fool w/ prompt design.”