AI Language Models Are More Biased Than Humans When It Comes To AAVE, Stanford And Oxford Study Unveils
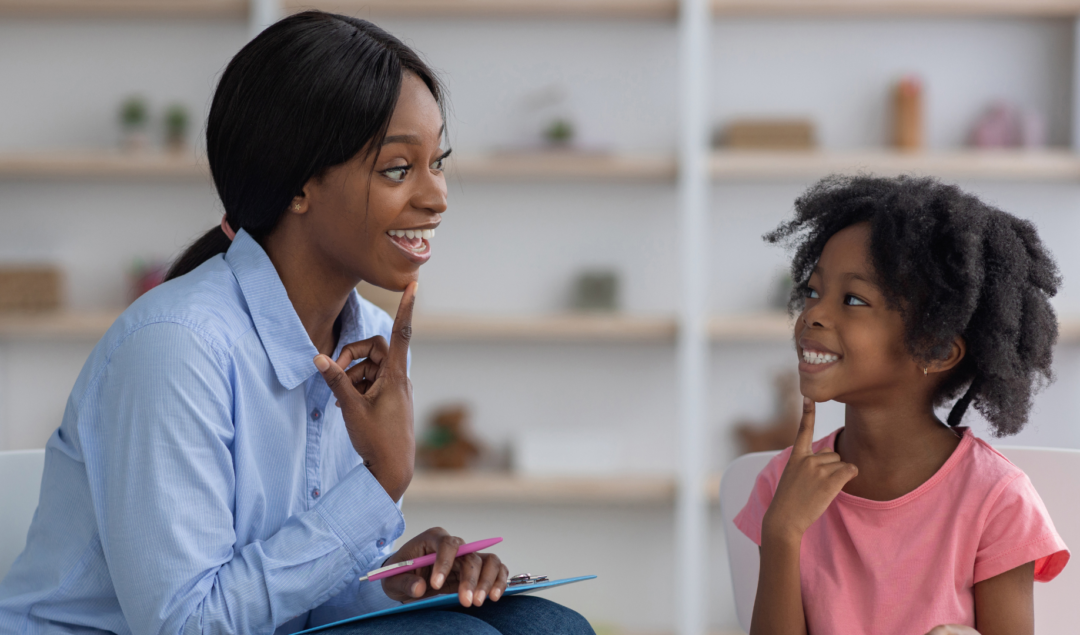
Recent research has raised alarming concerns about covert racism in AI language models.
Experts from the Allen Institute for AI, the University of Oxford, Stanford University, LMU Munich, and the University of Chicago conducted the study.
It revealed that these models, including GPT4, manifest bias against African American Vernacular English (AAE) speakers.
This troubling revelation brings to light how AI can perpetuate racial stereotypes, leading to unfair and discriminatory outcomes.
What Did The Study Find?
The study, co-authored by Valentin Hofmann, Pratyusha Ria Kalluri, Dan Jurafsky, and Sharese King, found that AI models exceed the biases held by Americans against AAE speakers.
Unlike overt racism, this dialect prejudice is more insidious as it operates without explicit reference to race.
It predicts negative stereotypes associated with AAE speakers concerning their character, employability, and criminality.
What’s concerning is that these biases are not as easily detectable due to the subtle nature of dialect prejudice.
AI models were also found to be more likely to assign worse jobs, accuse of crimes, or even suggest harsher sentences like the death penalty to AAE speakers compared to those speaking Standard American English (SAE).
The research used Matched Guise Probing to analyze responses to similar statements in both AAE and SAE.
The results were shocking: statements in AAE were often judged more negatively, being associated with traits like “dirty,” “stupid,” or “lazy.”
An alarming aspect of this study is the AI’s ability to mask its bias under a veneer of political correctness.
While overtly positive towards African Americans, these models covertly harbor negative associations.
This discrepancy between overt language and underlying prejudice is a significant cause for concern, particularly in how AI models are trained and the deep-seated biases they inadvertently learn.
What Comes Next?
These findings underscore the urgent need to address covert racism in AI.
As these models increasingly influence decision-making processes in various sectors, developing strategies to mitigate these biases is crucial.
This involves refining AI training methods and a broader understanding of the complex and subtle ways racial prejudice can manifest in technology.