Racial Bias and Gender Bias Examples in AI systems
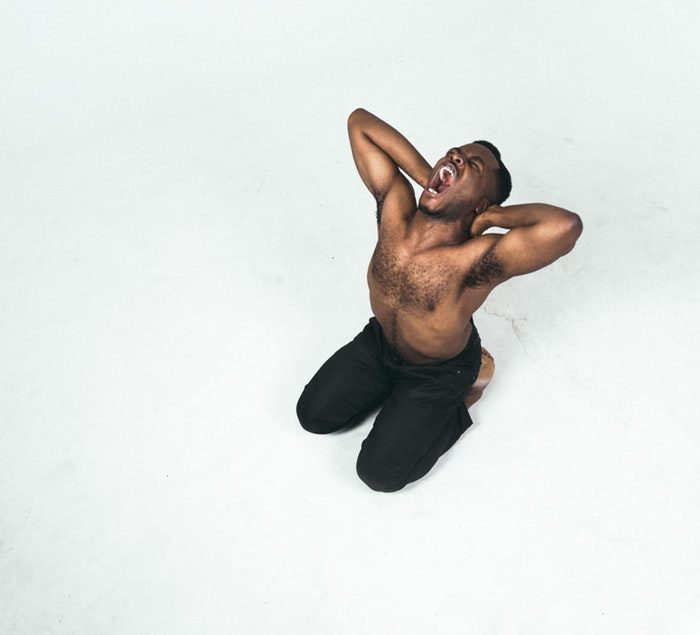
This article is a snippet from the postgraduate thesis of Alex Fefegha, the amazing technologist and founder of Comuzi. Here he breaks down concrete examples of racism and sexism perpetrated by A.I.
So here it goes:
Suggestions have made that decision-support systems powered by AI can be used to augment human judgment and reduce both conscious and unconscious biases. However, machine learning data, algorithms, and other design choices that shape AI systems may reflect and amplify existing cultural prejudices and inequalities. While historian of technology Melvin Kranzberg (1986) constructed the viewpoint that technology is regarded as neutral or impartial. A counter-argument is that AI systems could employ biased algorithms that do significant harm to humans which could go unnoticed and uncorrected until it is too late.
Racial Bias
ProPublica, a nonprofit news organization, had critically analyzed risk assessment software powered by AI known as COMPAS. COMPAS has being used to forecast which criminals are most likely to re-offend.
Guided by these risk assessments, judges in courtrooms throughout the United States would generate conclusions on the future of defendants and convicts, determining everything from bail amounts to sentences.
The software estimates how likely a defendant is to re-offend based on his or her response to 137 survey questions (an example of the survey is shown in fig. 1).
Figure 1: ’COMPAS Survey’, Julia Angwin et al. (2016). ProPublica compared COMPAS’s risk assessments for 7,000 people arrested in a Florida county with how often they reoffended (Angwin et al; 2016; Garber, 2016; Liptak, 2017).
It was discovered that the COMPAS algorithm was able to predict the particular tendency of a convicted criminal to re-offend. However, when the algorithm was wrong in its predicting, the results were displayed differently for black and white offenders.
Through COMPAS, black offenders were seen almost twice as likely as white offenders to be labeled a higher risk but not actually re-offend. While the COMPAS software produced the opposite results with whites offenders: they were identified to be labeled as a lower risk more likely than black offenders despite their criminal history displaying higher probabilities to re-offend (examples of results are shown in fig. 2–5).
Figure 2–5: ‘COMPAS Software Results’, Julia Angwin et al. (2016)
For context, to highlight the impact of a software such as COMPAS, the United States imprisoned 1,561,500 individuals in federal and state correctional facilities.
The United States imprisons more people than any country in the world, a large percentage of those imprisoned are black.
Race, nationality and skin color played a contributing role in composing such assessments and predictions until the 1970s when research studies uncovered implications leading to those attributes to be regarded politically unacceptable.
In 2014, the former U.S. Attorney General Eric Holder advised that the risk assessment scores are possibly implanting bias into courts environments.
Despite this discovery, the research study by ProPublica was disputed by a group of Ph.D. researchers. Their viewpoints were that the results by ProPublica contradict a number of existing studies concluding that risk assessment scores can be predicted free of racial and gender bias. The Ph.D. researchers’ conclusion of their research was that it is actually impossible for a risk score to satisfy both fairness criteria at the same time.
This is due to the developers of the COMPAS software, Northpointe refusing to disclose the details of its proprietary algorithm, making it impossible for researchers to assess the extent to which its algorithm may be unfair.
Revealing hidden information regarding their algorithm could limit Northpointe’s goal in being a competitive business. However, the action raises questions about government departments entrusting for-profit companies to develop risk assessment software of this nature.
In a court case, the Supreme Court of Wisconsin examined the validity of using the COMPAS risk-assessment software in the sentencing of an individual. The court case has been positioned by the media as one in the United States that has been a first in addressing concerns regarding a judge being assisted by an automated software-generated risk assessment score.
The Supreme Court ruled for the continued use of COMPAS to aid judges with sentencing decisions. However, the court expressed hesitation about the future use of the software in sentencing without information highlighting the limitations of COMPAS.
The court made a number of deliberations of its limitations:
1. COMPAS is a proprietary software, which the developers of the software have obviated the disclosure of explicit information about the impact of its risk factors or how risk assessment scores are calculated.
2. COMPAS risk assessment scores are established on group data, and therefore the software classified groups with characteristics that designate them as high-risk offenders but not high-risk individuals.
3. There has been a number of research studies which have proposed that the COMPAS algorithms develop biased results in how it analyzes black offenders.
4. COMPAS measures defendants/offenders to a national sample, but the software does not engage in a cross-validation study for a local population. Foresight potential issues as the COMPAS software must be regularly monitored and updated for accuracy as populations adjust.
5. The COMPAS software original purpose is not for sentencing but rather as an assistive tool in assessing an individual.
Gender Bias
In contrast to racial bias, there has been literature highlighted on its impact on the lives of humans in regards to algorithms being programmed into AI systems. Literature written about gender bias is still at the early stages, most of the content written about the topic are news articles that haven’t been backed with academic studies
An academic paper of interest which has led the debate regarding this topical area of concern is one titled Semantics derived automatically from language corpora contain human-like biases which was published in leading academic journal Science. There had been a number of research written about word embeddings and its applications from Web search to the dissection of keywords in CVs.
However, prior research did not recognize the sexist associations of word embeddings and its potential introduction of different biases into different software systems. The researchers employed a benchmark for documented human biases, the Implicit Association Test (IAT). The IAT has been adopted by numerous social psychology studies since its development.
As shown in figure 6–8, the test measures response times by human participants who are asked to pair word concepts displayed on a computer screen. Although the IAT has received criticism from academics in regards to the validity of its research findings, the test contributed a considerable role in the direction of this particular study.
Figure 6–8: ‘IAT Test Examples’, Taken from a number of sources.
The group of researchers constructed an experiment with a web crawler, which was programmed to function as an artificially intelligent agent participating in an Implicit Association Test.
The algorithm used in this experiment is similar to one that a startup technology company, who may be providing a service powered by AI which analyses CVs would employ at the core of its product.
The algorithm is able to produce co-occurrence statistics of words — words that often appear near one another have a stronger association than those words that rarely do.
The Stanford researchers employed the web crawler to act as an automatic indexer on a colossal fishing of contents from the internet, containing 840 billion words.
Once the indexing of information was completed, the group of researchers examined sets of target words while sifting through the extensive amount of content looking for data that would inform them of the potential biases humans can unwittingly possess.
Example words were ‘programmer, engineer, scientist, nurse, teacher and librarian’ while, the two sets of attribute words were man/male and woman/female.
In the results, the data highlighted biases such as the preference for ‘flowers over bugs’ (which could be illustrated as a harmless bias), however, the data identified bias along themes associated with gender and race.
A particular case is the autonomous intelligent agent associating feminine names more with words attributed with families such as ‘parents’ and ‘wedding’ than names of a masculine nature. On the other hand, masculine names had stronger associations with words attributed to a career such as ‘professional and salary’.
Caliskan et al make note that the learning experiment findings replicated the extensive proof of bias found in a number of previous Implicit Association Test studies, which has involved human participants. It could be suggested that these findings can be recognizable in objective reflections of how humans live.
There have been statistics highlighting unequal distributions of occupation types with respect to gender — The UK has the lowest percentage of female software engineering professionals in Europe, at less than 10% according to The Women’s Engineering Society (2018).
The project highlighted that the biases in the word embedding are in fact closely aligned with the social conception of gender stereotypes. Stereotypes have been described as both unconscious and conscious biases that are held among a group of people. A number of research studies have explored stereotypes playing a contributory position towards the data being used to train A.I.
What does the word “think” mean?
From an human-computer interaction perspective, when a technological innovation such as artificial intelligence is built into complex social systems such as criminal justice, health diagnoses, academic admissions, hiring, and promotion, it may reinforce existing inequalities, regardless of the intentions of the technical developers.
In regards to the ethical, questions develop such as what are ‘decisions’? How would an artificial system be able to make the ‘right’ choices to arrive at a calculated conclusion? What is the certain algorithmic instructions and data inputs that have been programmed into an artificial system giving it agency to judge and offer a verdict in the mannerisms of a human? In regards to A.I ethics, what does the word “think” really mean?