Recognizing Cultural Bias in AI
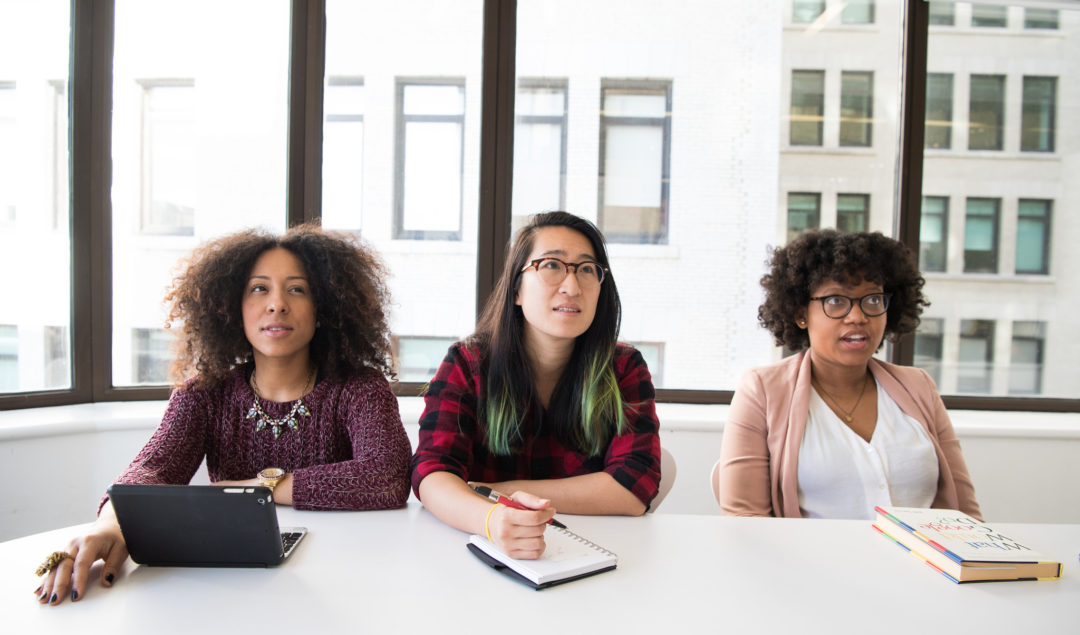
When tackling culture bias in Artificial Intelligence (AI), it is important to understand how much we use AI in our everyday lives. There are quite a few applications, and while they all have different names, a few of them are becoming more familiar to the general public. There are fields such as machine learning, face recognition, computer vision, virtual and augmented reality.
You can also find artificial intelligence in traffic lights, GPS navigation, MRIs, air traffic controller software, speech recognition, and robotics.
The point is, unlike the 90s, when AI was just starting to take off again, novice users are interacting with AI models on a daily and even hourly basis. So the question is what would happen if a service that you relied on for a day to day operation couldn’t see you?
MIT researcher Joy Buolamwini dealt with this quite literally. In her writing and videos, she talked about a time where she was completing research on social robots. She was using a 3D camera with facial recognition software. This software was unable to detect Joy’s face because of her darker skin tone. And it led to her wearing a white mask to complete her research. Eventually, Joy launched a website called the algorithmic justice league which looks at other ways cultural bias is entering the field of AI. She recently completed her master’s degree at MIT looking at the social implications of the technology and a TED Talk.
A study on one biased model from 2013 by Boston University and Microsoft has also been getting a lot of attention. In this study, they were looking at a training model called Word2Vec which was created by Google researchers. Its job is to build correlations and predictions for search results using word relationships. This model was based on the large database of Google news articles. Unfortunately, the training model proved that our language has a sexist basis. When they asked the model a question like, man is to computer programmer as a woman is to .. the answer was homemaker. There are other examples of the model’s sexist bias listed in the study.
This addresses a common question, why can’t we train AI models based on the internet to make them more inclusive? And the answer is, the internet is just as biased as we are. We are writing in these relationships. Similarly, the images you come across on the web are overwhelmingly represented with White people and not people of color. And so, facial recognition would also be affected by bias if trained only with data existing on the internet.
It is more apparent today that our behavior easily influences algorithms. It seems that bias is inherent in most of the actions we take. But this can be amplified in a model that does not have any filters on what is unacceptable behavior. One example is Microsoft’s past experiment with a machine learning model that was in charge of a Twitter account. They named it Tay, and its job was to respond to users interacting with it through tweets. It was quickly discovered that as more users tweeted off color remarks, Tay started to mirror and reciprocate those sentiments. The bot was quickly taken down and an apology issued.
Bias on the internet doesn’t stop with intentional acts to create a response to the one received from Tay, or the intention to harm at all. The recent election cycle has highlighted an issue in social media circulating ‘fake news’ or sensational news that spreads and spreads. Eli Pariser delivered a very interesting Ted Talk on what he calls filter bubbles. This where our biases live and never die or become re-informed. Eli gave an example of two different friends living in different places and placing a google search for Egypt. They both received different results based on their preferences. Search suggestions are often generated through algorithms also called recommendation algorithms, that are based on what users have clicked on and read in the past and their geo location. In his talk, Eli posed an interesting question, what if users were given tools to change the recommendation algorithm that was collecting and executing on their information. And give users the choice to allow information that challenges their worldview, focuses on local events, or the most popular events.
This touches on transparency in AI which leads us closer to a solution to our problem with cultural bias. What would you do if you knew that the reason you were seeing a particular Facebook post was not that of its validity but that it matches certain requirements of a model on your current preferences? Because the information we want to see is so readily available on our feeds, we might forget that behind the scenes are impersonal algorithms simply identifying content.
Recently, companies have taken to shutting down portions of their websites that yield unsavory results. For example, when Google Photos accidentally identified Black people as gorillas, in its attempt to automatically group the photos, the company’s response was to delete the tag gorilla so that nothing could be identified as a gorilla. LinkedIn also had an issue with suggestions of male names for female names. For example, a search for Stephanie might give results for Stephanie and Stephen but a search result for Stephen would not yield search results for Stephanie. In response to this LinkedIn removed the suggestion feature and only allowed the search to pull results based exactly on what was typed. But more transparency both in the development phase and on the user side can help identify these bugs early and allow users to understand the why an algorithm makes a certain choice.
There is a new technology on the block called Explainable AI or XAI. Its goal is to look at what happens in between a model identifying a goal and how much confidence is put into the model for its accuracy and success. For example, the image below contains a speed sign. Let’s say that to recognize this street sign the model breaks up the sign into smaller recognizable parts, like the border, strong lettering and font and the word speed and the number 5. It then makes a prediction based on the information it receives, which is either the right choice or the wrong choice. After looking at the results and assessing how many of the guesses were correct or not an accuracy is obtained.
The two middle columns of the figure above are what explainable AI does. It takes a look at WHY the algorithm made the choices it made. I recently heard a research from the University of Washington describe it this way. Their team built a model to distinguish huskies from wolves and correctly pick the right animal. Their model turned out to be pretty successful with a high accuracy. But with some more analysis, they discovered the model was looking at the snow around a wolf instead of the features of the animal itself.
Transparency and diversity are critical for the future of using AI in real life. But it does not just take diversity for programmers and developers. We also desperately need diversity in users. This is why I am a big fan of Google X’s Loon project that is helping more remote locations get online. Simply put, we need more data for our machine learning models, and we don’t have nearly enough. 100 samples for a data set might sound great, but 1,000 is even better.
Not everyone is a developer, but we are all users. Your basic internet use can help increase the inclusivity of the web. I encourage you to add your narratives online. By increasing the representation of voices that we don’t normally have access to we can change the stories we find on the web. By using more diverse stock photos, we can also increase the positive representation of minorities in media. And all of the new information we produce will get added to the models collecting word relationships and image searches. You are a part of the solution to make the world a better place!
If you would like to watch the video version of this article follow this link.