The Black And Brown Innovators That Made The MIT Under 35 List

Joelle Mbatchou, one of a few dozen people of color to make MIT’s Innovators Under 35 list this year, has done what some may describe as exceptional work over the years in the AI space.
The 32-year-old has developed a machine-learning model called Regenie that makes analyzing the data quicker and cheaper while reducing the amount of computing power required.
The method could allow researchers to identify genetic variants associated with specific diseases more easily. “With the increasing number of collaborations being established across large biobanks, many of them involving individuals from diverse populations, Regenie makes it possible to leverage those data and … potentially make discoveries which can lead to improved clinical care,” she says.
Here we’ve listed some of the Black and Brown innovators that made the MIT Innovators Under 35 2022 List.
Kathleen Siminyu
Advances in speech and language technologies have led to tools like voice-enabled search, text-to-speech apps, speech recognition, and machine translation, but such tools only work for the languages they’ve been trained to recognize—typically English, French, or Chinese.

For many other languages, including ones spoken by millions of Africans, they remain out of reach. Kathleen Siminyu, 28, wants to change that. She launched a fellowship program through which contributors created nine open-source African-language data sets annotated for a variety of machine-learning tasks. She sees “a possible future where all the information readily available on the internet is equally accessible in African languages as it is in English.”
Setor Zilevu
Previously, 27-year-old Setor Zilevu worked at Google as a User Experience Contractor. His research focused on understanding growth and monetization for Google Meet.
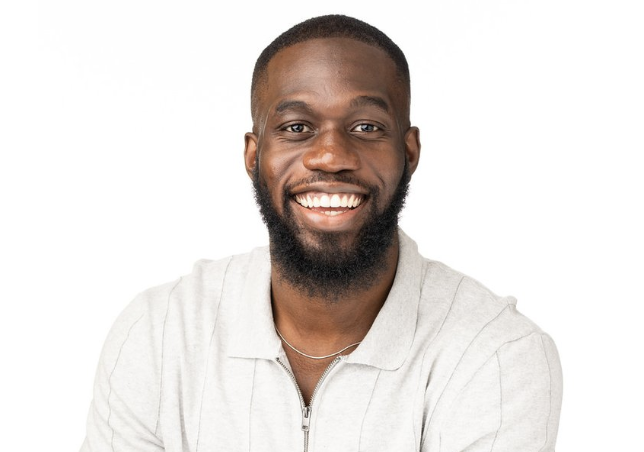
He graduated from Virginia Tech with a Ph.D. in Computer Science, and his dissertation focused on Human-Computer Interaction (HCI) for Complex Machine Learning.
Additionally, Zilevu designed and evaluated interfaces to understand human behavior and human movement performance for machine learning systems in assisting stroke survivors with in-home therapy.
In Summer 2020, Zilevu interned at Facebook as a User Experience Researcher. His work included using qualitative methods to increase user engagement and retention for Workplace. Additionally, in summer 2019, he interned at Adobe Corporation on the Digital Media Experience team, working as a design engineer.
Gauri Joshi
State-of-the-art machine-learning projects often require massive amounts of data and computational power. As a consequence, only a few groups with these resources control access to many machine-learning models. Gauri Joshi, 34, is working to change that by designing distributed computing algorithms that make it possible for such models to be trained using a network of devices such as cell phones or sensors. “It democratizes machine learning and makes it universally accessible without requiring expensive computing hardware and enormous amounts of training data,” Joshi says.

Uzoma Orchingwa
Uzoma Orchingwa co-founded Ameelio, a nonprofit that includes a website and an app to support the flow of information and correspondence between incarcerated people and their loved ones on the outside—all for free.
Why such a need? Because in jails and prisons, everything costs more than on the outside. A single phone call? Up to $25. Video calls? As high as $1 per minute. An email? Thirty cents to a dollar.

Until Ameelio, there wasn’t a free, not-for-profit alternative to prison communications that millions of people depend on every single day. It’s a business that generates approximately $1.2 billion per year and profits mainly two companies.
Ishan Misra
To be accurate, many AI models need large amounts of human-labeled data. Research from Ishan Misra, 31, shows that it’s possible to train these models on visual data alone, skipping the human labels. Misra believes that such self-supervised models will greatly expand the types of problems that AI can solve.

“In domains like medical imaging, where labeling is expensive, self-supervised models can play a major role in rapidly developing AI models at a fraction of the cost,” he says.
“These models can also enable AI models to learn new skills continuously from the stream of data they observe, without human supervision.”
That could be especially useful for robots operating in environments that constantly change.
The full list is available here: MIT Technology Review.